Part of this project was in collaboration with NASA JPL MAARS: Machine learning-based Analytics for Rover Systems.
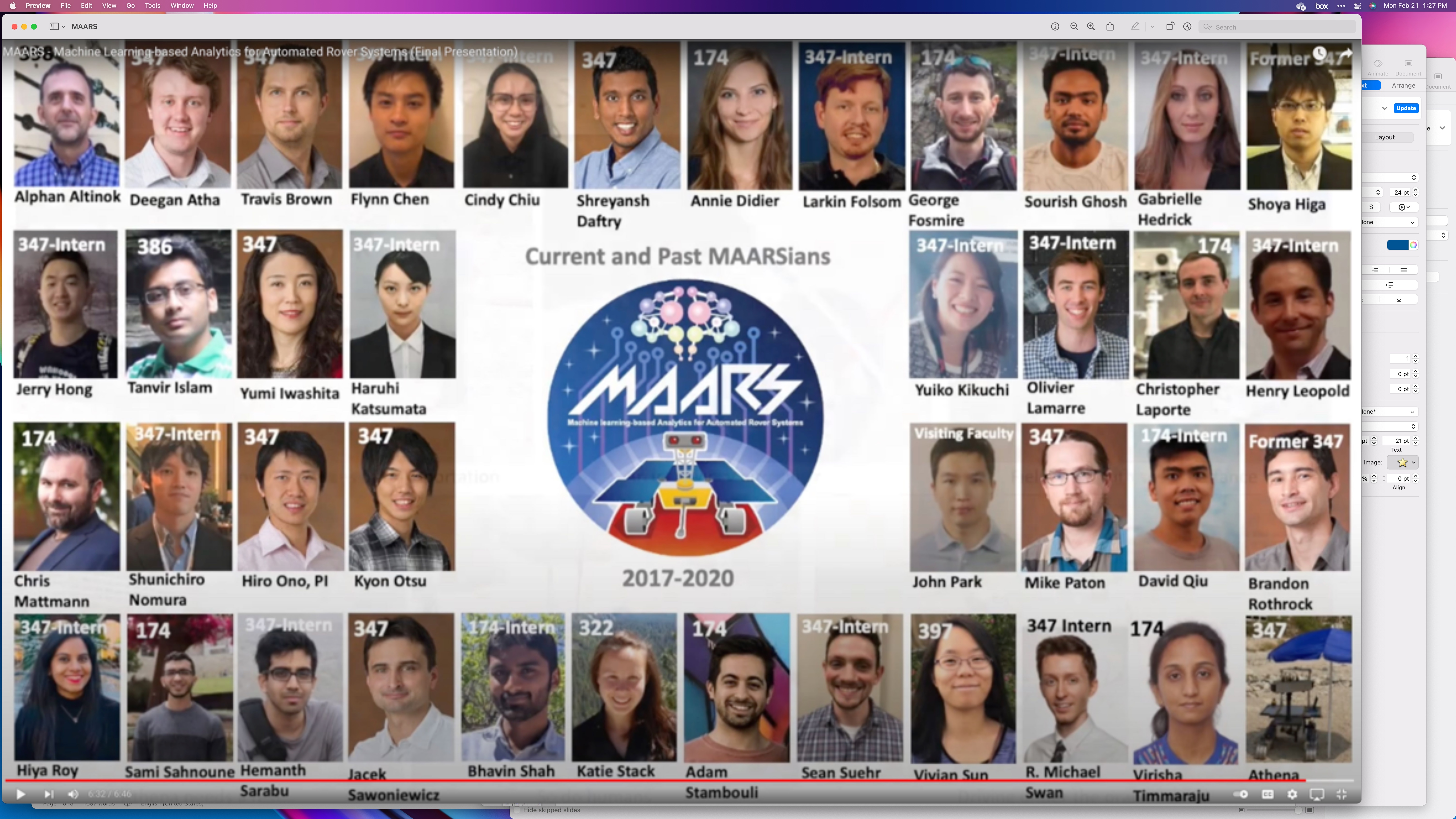
Imagine you are a food writer for a magazine, sent to a city for a day to discover and report on local cuisine. You have three meals (breakfast, lunch, and dinner) over which to gather information and maximize your visit to create the clearest impression for your readers. As you come into town, you have a decision to make. Will you visit familiar, fast food and chain restaurants, or, will you seek out new, undiscovered eateries to paint your culinary picture? Obviously, patronizing unfamiliar restaurants is going to yield new, quality information, helping readers back at home draw sound, comprehensive conclusions about the locale.
Following the same logic, imagine navigating with a Google map. The most direct route has a reputation for heavy traffic which could jeopardize timely arrival. A slightly longer distance with less traffic might give you a better chance to reach your destination on time. Maximizing information gain by traveling unchartered territory is the goal of Dr. John Park’s research. Park is using autonomous and connected vehicles armed with high-performance onboard computers to traverse unexplored areas. Under strict limitations of time and energy, he is trying to gain and transmit the most data possible from new, dynamic environments.
On the Floor
“We are not vacuuming, it just looks like we want a (very!) clean rug,”
says Park. Five Ph.D. candidates are kneeling on the floor, encircling a test-bed in the middle of Park’s Hines Hall lab. The team has taped-out a grid on low-pile carpet to border a series of two by two-foot squares called “cells”. Crawling over the carpet cells are four iRobot Roomba® vacuums.
While most Consumers purchase these devices in a pre-programmed suction mode, Park acquired them unprogrammed. His team has fitted each Roomba® with two round decks creating tiered towers above each device. The hip, techy, blinking wedding cakes crawl and spin on the floor, learning, updating, clarifying and confirming their environment. “The process by which autonomous vehicles learn about carpet in Hines Hall using is actually quite similar to how we would receive and process data in other, more difficult environments,” explains Park. “Autonomous vehicles allow operators to observe, record, make decisions and even take action in locations where physical human presence is either impossible or undesirable.”
Adorning the Roomba decks are serious technology: ZED Stereo Cameras, RPLIDAR 360-Degree Laser Ranger Scanners, NVIDIA Jetson AGX Xavier High-Performance Computing Units and Cray Supercomputers. Funding for this and other equipment has been provided by the NASA Jet Propulsion Laboratory, the Virginia Department of Transportation, the North Carolina Department of Transportation, the United States Department of Transportation, and most recently a $240,000 three-year grant from the National Science Foundation. This new project is known as IMPACT (Information-theoretic Multiagent Paths for Anticipatory Control of Tasks).
Learn, Update, Clarify, Confirm
Park’s project promotes the scientific and engineering value of intelligent navigation systems by finding the best routes of vehicles with autonomous decision-making based on the desired level of exploration, risk, and energy constraints. The navigation analyzes images (autonomous driving feature detection), selectively collecting data without interrupting their trips.
When an autonomous vehicle travels through Park’s floor grid, information is gained by visiting unclassified or uncertainly classified cells, observing the condition in those cells, and estimating the entropy (degree of disorder or randomness) in other cells. Each vehicle updates its path plan every time it moves to a new cell. By sharing information about the state of the cells it encounters, it helps to define the optimal parameters to be used in other vehicles’ journeys. If a cell is visited by another vehicle and found to be in the same state as the original cell of that type, then all vehicles have confirmation that these cells are correlated. The vehicles gather and confirm data during their journeys, updating Park’s knowledge bank as new information about the terrain is discovered.
Understanding how information can be learned throughout navigation will produce a guide to government planners and transportation engineers, and offer substantial benefits to society in improved choice modeling especially in congested traffic networks when heterogeneous users cause complex situations with weak road resilient networks. Future navigation and autonomous vehicle driving will realize improved efficiency by considering the tradeoffs between energy, time and environmental challenges.
The Team
Dr. Park has staffed his grant with five capable Ph.D. candidates from the Department of Computational Science and Engineering in the College of Engineering. Khadijeh Shirzad, Justice Darko, Yaa Takiwaa Acquaah, Larkin Folsom, and Nigel Pugh are fearless, despite the enormous programming tasks ahead. While they describe this project as “intricate” and “complicated” the team seems clear-minded and process-oriented with how they intend to gather and analyze the robots’ data.
The five students hail from three countries boasting majors in mathematics, physics and computer science, yet all seem to agree on one thing: while computational science and engineering is a complex, multifaceted field, it lives in reality.
“Our studies mimic and therefore benefit real life,”
says Acquaah.
“We use math and computational thinking to analyze data, solve pressing problems, and improve the world.”
written by Kelly S. Morgan
Chancellor Martin recognizes work.





